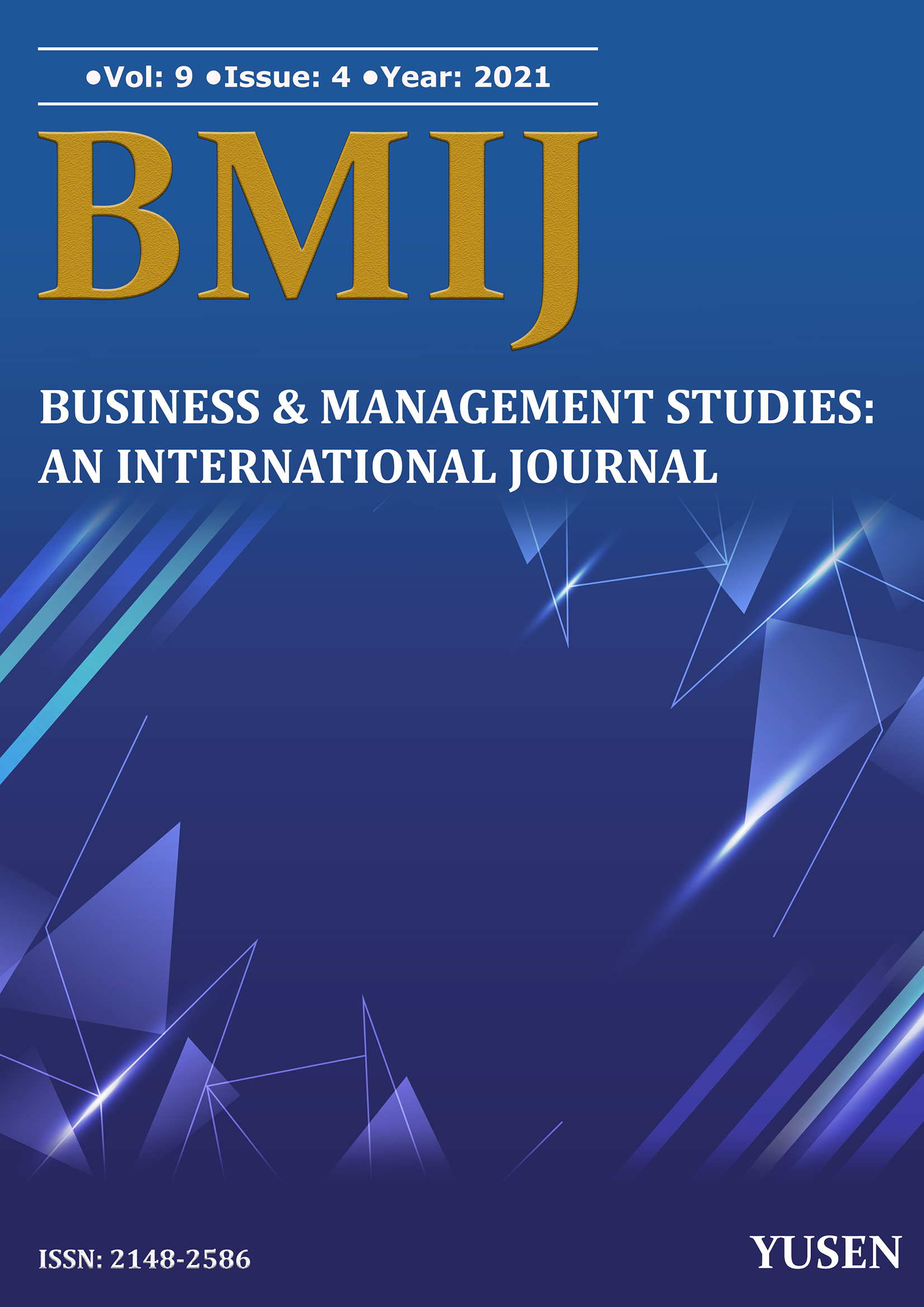
Yayınlanmış 2021-12-25
Nasıl Atıf Yapılır
Telif Hakkı (c) 2021 İbrahim Sabuncu- Eda Şen

Bu çalışma Creative Commons Attribution-NonCommercial-NoDerivatives 4.0 International License ile lisanslanmıştır.
Özet
Bu çalışmanın amacı, sosyal medya verilerinden yararlanarak politikacıların oy oranlarının günlük değişiminin ve seçim sonucunun tahmin edilebilirliğinin araştırılmasıdır. Bu amaçla yapılan çalışmada, 3 Kasım 2020 ABD seçimine katılan adaylar hakkında 01.07.2020- 03.11.2020 tarihleri arasında paylaşılan 20.746.834 adet tweet RapidMiner programı ile Twitter platformundan toplanmıştır. Twitter’dan toplanan verilere, Vader algoritması ile duygu analizleri yapılmıştır. Tweetler, pozitif, negatif, NPS (pozitif-negatif) ve nötr duygu kategorilerine göre gruplandırılmıştır. Duygu kategorilerine ayrılmış tweet sayıları kullanılarak, günlük oy oranlarını ve seçim sonucunu tahmin etmek için altı farklı makine öğrenmesine dayalı tahmin modeli oluşturulmuştur. Tahmin modellerinde, bağımsız değişkenler adaylar hakkında paylaşılan duygu kategorilerine göre ayrılmış günlük Twitter verisidir. Bağımlı değişkenler ise anket ve ekonomik göstergelere dayalı yapılmış, adayların günlük oy oranı tahminleridir. Tahmin modelleri 109 günlük veri ile eğitilmiştir. En doğru sonucu veren tahmin modeli Derin Makine Öğrenmesi (Deep Machine Learning) algoritması kullanılarak, seçim sonucu %1,7 hata payıyla tahmin edilebilmiştir. Bu çalışma, Twitter’daki çok çeşitli manipülasyonlara rağmen, makine öğrenmesi aracılığıyla, Twitter’ın halen politik eğilimlerin takibi ve seçim sonuçları tahmininde kullanılabilecek bir veri kaynağı olabileceğini göstermektedir.
İndirmeler
Referanslar
- Bansal, B. ve Srivastava, S. (2018). On predicting elections with hybrid topic based sentiment analysis of tweets. Procedia Computer Science, 135, 346–353. doi:10.1016/j.procs.2018.08.183
- Burnap, P., Gibson, R., Sloan, L., Southern, R. ve Williams, M. (2016). 140 characters to victory?: Using Twitter to predict the UK 2015 General Election. Electoral Studies, 41, 230–233. doi:10.1016/j.electstud.2015.11.017
- Canipe, C., Levine, A. J. ve Hart, S. (2020). U.S. election results. 18 Şubat 2021 tarihinde https://graphics.reuters.com/USA-ELECTION/RESULTS-LIVE-US/jbyprxelqpe/ adresinden erişildi.
- Castro, R., Kuffó, L. ve Vaca, C. (2017). Back to #6D: Predicting Venezuelan states political election results through Twitter. 2017 4th International Conference on eDemocracy and eGovernment, ICEDEG 2017, 148–153. doi:10.1109/ICEDEG.2017.7962525
- Cerf, V. G. (2017). Information and misinformation on the Internet. Commun. ACM(CACM), 60(1), 9. doi:10.1145/3018809
- Ceron Guzman, J. A. (2016). A Sentiment Analysis Model of Spanish Tweets.
- Chatfield, A., Reddick, C. ve Choi, K. (2017). Online Media Use of False News to Frame the 2016 Trump Presidential Campaign. doi:10.1145/3085228.3085295
- Conway, B. A., Kenski, K. ve Wang, D. (2015). The rise of Twitter in the political campaign: searching for intermedia agenda-setting effects in the presidential primary. J. Comput. Mediat. Commun., 20(4), 363–380.
- Economist, T. (2020). Forecasting the US elections. 12 Aralık 2020 tarihinde https://projects.economist.com/us-2020-forecast/president adresinden erişildi.
- Golbeck, J., Grimes, J. M. ve Rogers, A. (2010). Twitter use by the U.S. Congress. J. Am. Soc. Inf. Sci. Technol., 61(8), 1612–1621.
- Golovchenko, Y., Buntain, C., Eady, G., Brown, M. A. ve Tucker, J. A. (2020). Cross-platform state propaganda: Russian trolls on Twitter and YouTube during the 2016 US presidential election. The International Journal of Press/Politics, 25(3), 357–389.
- Graham, T., Jackson, D. ve Broersma, M. (2016). New platform, old habits? Candidates use of Twitter during the 2010 British and Dutch general election campaigns. Sage Journals, 18(5), 765–783.
- Grover, P., Kar, A. K., Dwivedi, Y. K. ve Janssen, M. (2019). Polarization and acculturation in US Election 2016 outcomes – Can twitter analytics predict changes in voting preferences. Technological Forecasting and Social Change, 145(September), 438–460. doi:10.1016/j.techfore.2018.09.009
- Jamieson, K. H. (2020). Cyberwar: how Russian hackers and trolls helped elect a president: what we don’t, can’t, and do know. Oxford University Press.
- Karami, A., Lundy, M., Webb, F., Turner-McGrievy, G., McKeever, B. W. ve McKeever, R. (2021). Identifying and analyzing health-related themes in disinformation shared by conservative and liberal Russian trolls on twitter. International journal of environmental research and public health, 18(4), 2159.
- Kelly Garrett, R. ve Weeks, B. E. (2013). The promise and peril of real-time corrections to political misperceptions. Proceedings of the ACM Conference on Computer Supported Cooperative Work, CSCW, (February 2013), 1047–1057. doi:10.1145/2441776.2441895
- Kim, A. J. ve Ko, E. (2010). Impacts of luxury fashion brand’s social media marketing on customer relationship and purchase intention. J. Glob. Fash.Mark., 1(3), 164–171.
- Kušen, E. ve Strembeck, M. (2018). Politics, sentiments, and misinformation: An analysis of the Twitter discussion on the 2016 Austrian Presidential Elections. Online Social Networks and Media, 5, 37–50. doi:10.1016/j.osnem.2017.12.002
- Makazhanov, A., Rafiei, D. ve Waqar, M. (2014). Predicting political preference of Twitter users. Social Network Analysis and Mining, 4(1), 1–15. doi:10.1007/s13278-014-0193-5
- RapidMiner. (2020a). Generalized Linear Model. 6 Kasım 2020 tarihinde https://docs.rapidminer.com/latest/studio/operators/modeling/predictive/functions/generalized_linear_model.html adresinden erişildi.
- RapidMiner. (2020b). Deep Learning. 6 Kasım 2020 tarihinde https://docs.rapidminer.com/latest/studio/operators/modeling/predictive/neural_nets/deep_learning.html adresinden erişildi.
- RapidMiner. (2020c). Decision Tree. 6 Kasım 2020 tarihinde https://docs.rapidminer.com/latest/studio/operators/modeling/predictive/trees/parallel_decision_tree.html adresinden erişildi.
- RapidMiner. (2020d). Random Forest. 6 Kasım 2020 tarihinde https://docs.rapidminer.com/latest/studio/operators/modeling/predictive/trees/parallel_random_forest.html adresinden erişildi.
- RapidMiner. (2020e). Gradient Boosted Trees. 6 Kasım 2020 tarihinde https://docs.rapidminer.com/latest/studio/operators/modeling/predictive/trees/gradient_boosted_trees.html adresinden erişildi.
- RapidMiner. (2020f). Support Vector Machine. 6 Kasım 2020 tarihinde https://docs.rapidminer.com/latest/studio/operators/modeling/predictive/support_vector_machines/support_vector_machine.html adresinden erişildi.
- Sabuncu, İ. (2020). USA Nov.2020 Election 20 Mil. Tweets (With Sentiment And Party Name Labels) Dataset. 20 Kasım 2020 tarihinde https://ieee-dataport.org/open-access/usa-nov2020-election-20-mil-tweets-sentiment-and-party-name-labels-dataset adresinden erişildi.
- Toker, H., Erdem, S. ve Özşarlak, P. (2017). 2015 Haziran Ve Kasım Seçimlerinde Siyasal Eğilim: Yeni Bir Kamuoyu Ölçümleme Aracı Olarak Twitter. Erciyes İletişim Dergisi, 5(1), 221–234.
- Tumasjan, A., Sprenger, T. O., Sandner, P. G. ve Welpe, I. M. (2010). Predicting elections with twitter: What 140 characters reveal about political sentiment. Fourth international AAAI conference on weblogs and social media içinde (C. 37, ss. 455–479). Citeseer. doi:10.15581/009.37.2.455-479
- Ulusoy, N. (2012). Sözlüklerdeki Sinema Sevgisi: New York’ta Beş Minare ve Çoğunluğun İnternet Sözlüklerine Yansıması. Beta Yayıncılık, İstanbul, 195–211.
- Wicaksono, A. J., Suyoto ve Pranowo. (2017). A proposed method for predicting US presidential election by analyzing sentiment in social media. Proceeding - 2016 2nd International Conference on Science in Information Technology, ICSITech 2016: Information Science for Green Society and Environment, 276–280. doi:10.1109/ICSITech.2016.7852647