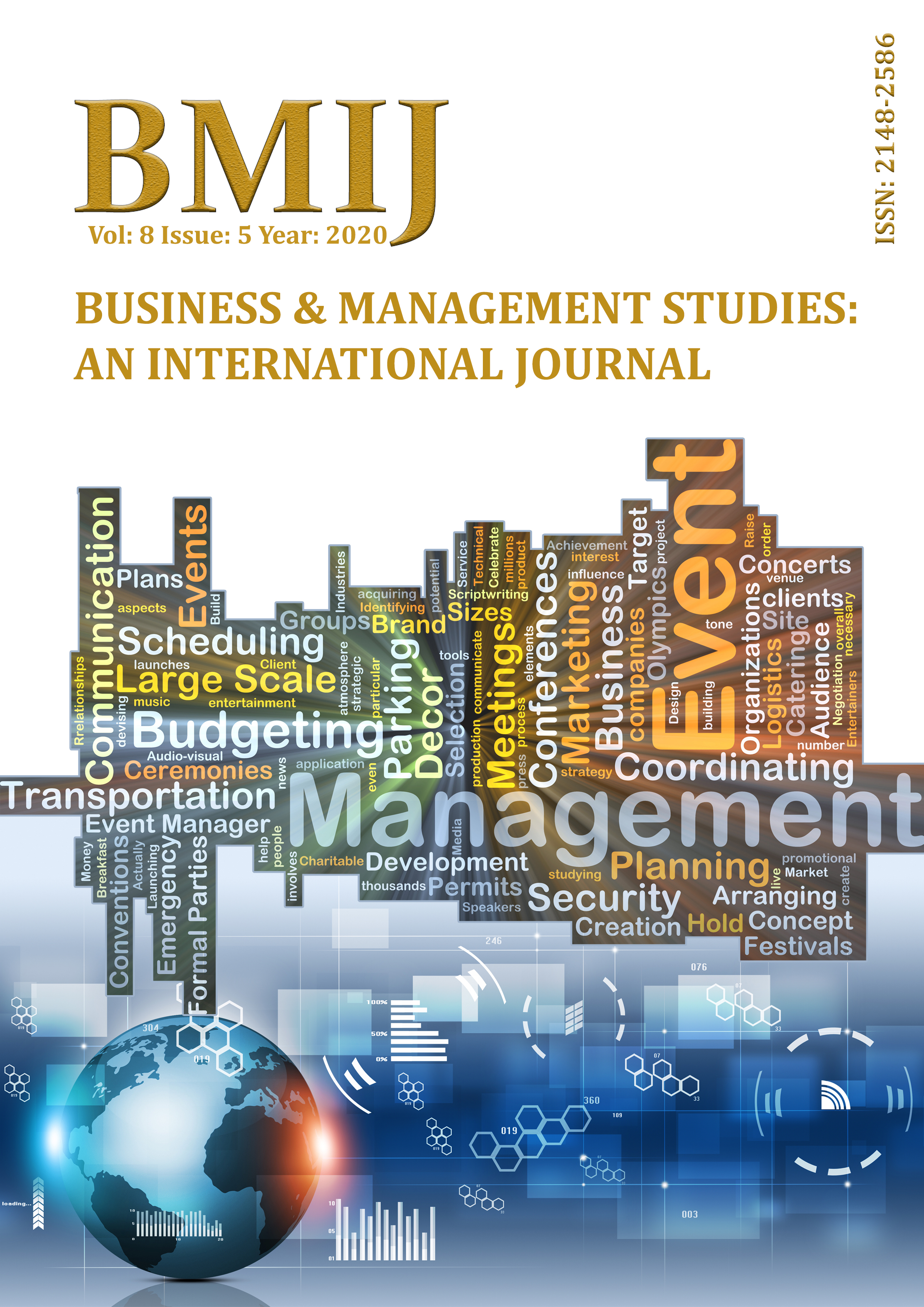
Yayınlanmış 25.12.2020
Anahtar Kelimeler
- Volatility, BIST Banking Index, ARCH, GARCH
- Volatilite, BIST Banka Endeksi, ARCH, GARCH
Nasıl Atıf Yapılır
Telif Hakkı (c) 2020 Business & Management Studies: An International Journal

Bu çalışma Creative Commons Attribution-NonCommercial-NoDerivatives 4.0 International License ile lisanslanmıştır.
Nasıl Atıf Yapılır
Öz
Bu çalışmada, 4 Ocak 2010 ile 31 Aralık 2019 dönemi arasında yer alan BIST Banka endeksine ait günlük kapanış verilerden hareketle endeksin volatilite modellemesi tahmin edilmiştir. Bu bağlamda, öncelikle fiyat serisinin Augmented Dickey-Fuller birim kök testi yardımıyla durağanlığı araştırılmış ve birinci dereceden durağan bir seri olduğu tespit edilmiştir. Daha sonra otoregresif modeller denendikten sonra en iyi ortalama denklem modelinin ARMA (2,2) olduğu saptanmıştır. Bunun yanında ortalama denkleme ait hata terimlerinde ARCH etkisi olduğu gözlemlenmiş ve buradan hareketle BIST Banka endeks serisinin hangi koşullu varyans modeli ya da modelleri ile açıklanabileceği test edilmiştir. Elde edilen test sonuçlarına göre BIST Banka serisinin volatilite modellemesini tahmin etmede en iyi sonuçlar veren modelin bilgi kriterlerine göre kıyaslandığında TGARCH (0,1,1); öngörü performansına göre kıyaslandığında ise EGARCH (1,1,1) modeli olduğu tespit edilmiştir.
Referanslar
- Birau, R., Trivedi, J. & Antonescu, M. (2015). “Modelling S&P Bombay Stock Exchange BANKEX Index Volatility Patterns Using GARCH Models”. Procedia Economics and Finance, 32(1), 520-525.
- Bollerslev, T. (1986). “Generalized Autoregressive Conditional Heteroskedasticity”.Econometrica. 31(3), 307-327.
- Çelik, İ., Özdemir A. & Gülbahar Demir S. (2018). "İslami Hisse Senedi Endeksleri Arasında Getiri ve Volatilite Yayılımı: Gelişmiş ve Gelişmekte Olan Piyasalarda Çok Değişkenli VAR-EGARCH Uygulaması" Muhasebe ve Finans İncelemeleri Dergisi. 1(2), 89-100.
- Çil, N. (2018). "Finansal Ekonometri".Der Yayınları, İstanbul.
- Dickey, D. A., & Fuller, W. A. (1981). “Likelihood Ratio Statistics for Autoregressive Time Series With A Unit Root”. Econometrica, 49(4), 1057-1072.
- Engle, R. F. (1982). “Autoregressive Conditional Heteroscedasticity with Estimates of the Variance of United Kingdom Inflation”. Econometrica. 50(4),987–1007.
- Jarque, C. M. & Bera, A. K. (1980). “Efficient Tests for Normality, Homoscedasticity and Serial Independence of Regression Residuals”. Economics Letters, 6, 255-259.
- Kalaycı, Ş. (2005). "Borsa ve Ekonomide Volatilite İlişkisi: İMKB’de Bir Şartlı Varyans Analizi" Süleyman Demirel Üniversitesi İktisadi ve İdari Bilimler Fakültesi Dergisi, 10(1), 241-250.
- Karahanoğlu, I., & Ercan, H. (2015). "BNK10 Endeksindeki Kaldıraç Etkisinin Genelleştirilmiş Otoregresif Koşullu Değişen Varyans Modeli ile Analiz Edilmesi". Uluslararası Alanya İşletme Fakültesi Dergisi, 7(3), 169-181.
- Koy, A. (2016). "Borsa İstanbul Sektör Endekslerinin Volatilite Modellemesi". Trakya Üniversitesi İİBF E-Dergi, 5(2), 1-23.
- Köseoğlu, S. D. (2010). "1997-2010 Dönemi Türk Bankacılık Sektörü Risk Analizi". Niğde Üniversitesi İİBF Dergisi, 3(2), 119-134.
- Kula, V. & Baykut, E. (2017). "BIST Banka Endeksi’nin (XBANK) Volatilite Yapısının Markov Rejim Değişimi GARCH Modeli (MSGARCH) ile Analizi". Bankacılar Dergisi, (102), 89-110.
- Kula, V. & Baykut, E. (2018). "BIST Şehir Endekslerinin Volatilite Yapıları ve Rejim Değişimlerinin Analizi" Muhasebe ve Finans İncelemeleri Dergisi. 1(1),38-59.
- Kuzu, S. (2018). "Borsa İstanbul Endeksi (BIST 100) Getiri Volatilitesinin ARCH ve GARCH Modeli ile Tahmin Edilmesi" Muhasebe ve Vergi Uygulamaları Dergisi. Özel Sayı, 608-624.
- Nelson, D. B. (1991). “Conditional Heteroskedasticity in Asset Returns: A New Approach”. The Econometric Society. 59(2), 347-370.
- Sevüktekin, M., & Nargeleçekenler, M. (2010). "Ekonometrik Zaman Serileri Analizi". Ankara: Nobel Yayıncılık.
- Tamilselvan, M. & Shaik, M. V. (2016). “Forecasting Stock Market Volatility – Evidence From Muscat Security Market Using GARCH Models”.International Journal of Commerce and Finance, 2(1), 37-53.
- Tuna, K. & İsabetli, İ. (2014). "Finansal Piyasalarda Volatilite ve BIST-100 Örneği" Kocaeli Üniversitesi Sosyal Bilimler Dergisi, (27), 21-31.
- Zakoian, J. M. (1994). “Threshold Heteroskedastic Models”.Journal of Economic Dynamics and Control, 18(5), 931-955.